The Importance of Image Annotation for Machine Learning in Business
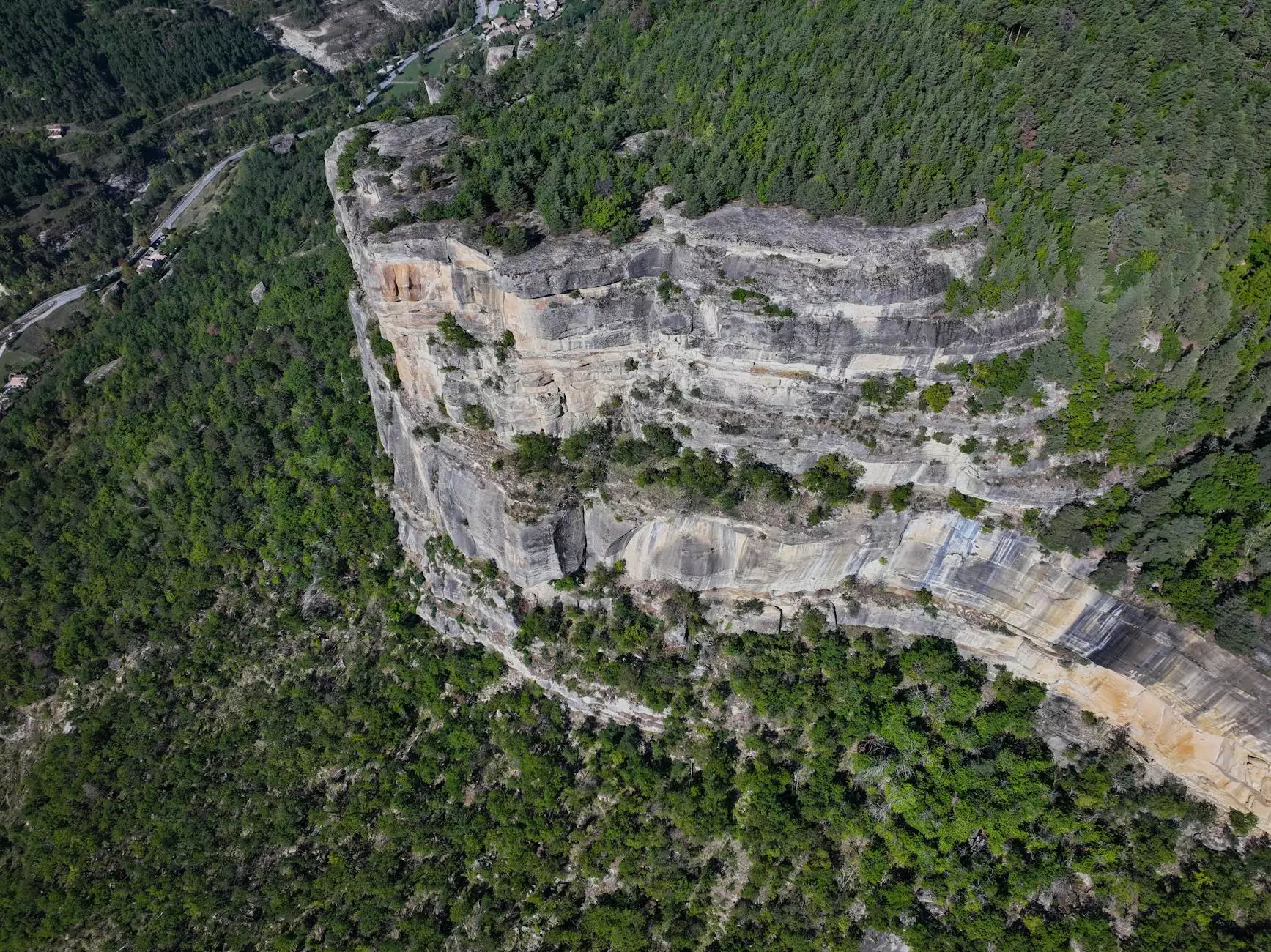
In today’s digital landscape, the role of image annotation for machine learning cannot be overstated. As businesses increasingly turn to artificial intelligence to enhance their operations, the demand for high-quality annotated data is more critical than ever. Image annotation provides the groundwork for machine learning models, enabling them to recognize patterns, generate insights, and improve decision-making processes.
Understanding Image Annotation
Image annotation is the process of labeling images to create a dataset that machine learning algorithms can learn from. This involves assigning tags or metadata to images, such as identifying objects, classifying scenes, and highlighting important features. These labeled images are essential for training models to recognize and understand visual data.
Types of Image Annotation
Different types of image annotation techniques are utilized in machine learning, each serving specific purposes. Here are some common methods:
- Bounding Box Annotation: This involves drawing rectangles around objects within an image to help the model learn the object’s position and size.
- Semantic Segmentation: Here, each pixel of the image is classified, allowing for precise object detection and recognition at a pixel level.
- Polygon Annotation: This method is used for irregularly shaped objects in images, providing greater accuracy than bounding boxes.
- 3D Cuboid Annotation: Used primarily in autonomous vehicle applications, this technique annotates 3D objects in space to understand their volume and position.
The Business Implication of Image Annotation
The intersection between image annotation for machine learning and business is profound, impacting various sectors such as retail, healthcare, automotive, and more. Let's explore how this technology is redesigning business paradigms.
Enhancing Product Recognition in Retail
In the retail sector, image annotation helps businesses improve their product recognition capabilities. By annotating images of products, businesses can train machine learning models that power visual search engines. Customers can upload images and find similar items, thereby enhancing their shopping experience. Implementing this technology can lead to increased sales and customer satisfaction.
Revolutionizing Healthcare Diagnostics
In healthcare, the accuracy of medical imaging is paramount. Image annotation enables the training of machine learning models that assist in diagnosing diseases from imaging data such as MRIs, CT scans, and X-rays. By annotating images with labels indicating disease presence or absence, healthcare AI systems can learn to identify critical features, thus facilitating quicker and more accurate diagnoses.
Improving Autonomous Vehicle Navigation
For companies in the automotive industry, particularly those focused on autonomous vehicles, image annotation is essential for creating safe AI-driven navigation systems. Annotating road signs, vehicles, pedestrians, and various other environmental factors allows these systems to interpret their surroundings accurately, leading to improved safety and efficiency in vehicle operation.
The Process of Image Annotation: From Raw Data to Annotated Images
Effectively implementing image annotation for machine learning requires a systematic approach. Below are key steps in the annotation process:
1. Data Collection
The first stage involves gathering the raw images that need to be annotated. These images could come from various sources such as cameras, drones, or image databases.
2. Choosing Annotation Tools
Several tools and software are available for image annotation. Depending on project specifications, businesses can select from various platforms ranging from simple ones requiring fewer features to more robust, advanced tools that support complex annotation tasks.
3. Annotation Process
This is where the actual labeling happens. Depending on the chosen method (bounding boxes, polygons, etc.), human annotators or automated systems label the images. It’s crucial to ensure consistency and accuracy during this process to maintain data integrity.
4. Quality Assurance
A critical step that cannot be overlooked is quality assurance. Reviewing annotated images for accuracy and consistency helps prevent errors that could negatively impact machine learning models' performance.
5. Model Training
Once the annotated dataset is confirmed for quality, it can be used to train machine learning models. These models learn to recognize patterns and make predictions based on the provided labeled data.
The Benefits of Investing in Image Annotation
Businesses that invest in high-quality image annotation for machine learning can reap numerous benefits, including:
- Increased Accuracy: Well-annotated datasets contribute to higher accuracy in machine learning models, leading to improved outputs.
- Faster Time to Market: Automating image annotation processes can significantly reduce the time taken to prepare data for model training and deployment.
- Cost-Effectiveness: Outsourcing annotation tasks or leveraging automated tools can be a cost-effective strategy for businesses, especially for those with large datasets.
- Scalability: As businesses grow, having a robust image annotation process allows them to scale their machine learning efforts without compromising on data quality.
Challenges in Image Annotation
Despite its benefits, there are challenges associated with image annotation for machine learning. These include:
1. High Labor Costs
Image annotation can be labor-intensive, requiring significant time and effort to ensure quality, especially when human annotators are involved.
2. Subjectivity in Annotation
In certain cases, the interpretation of images can be subjective. Different annotators might label the same image differently, leading to inconsistencies in the training data.
3. Complexity of Images
Not all images contain clear or distinct objects, which can complicate the annotation process. Complex scenes with multiple overlapping objects can pose challenges.
Future of Image Annotation in Business
The landscape of image annotation for machine learning is evolving rapidly. Technical advancements, including automated annotation tools and improved machine learning algorithms, are transforming the way businesses approach image data. As these technologies mature, we can expect:
- Increased Automation: More businesses will utilize automated annotation tools to minimize human error and reduce costs.
- AI-Enhanced Annotation: Machine learning models themselves will be used to assist or even take over the annotation process, improving speed and efficiency.
- Growth in Industry-Specific Solutions: Tailored annotation solutions for specific industries, such as healthcare, automotive, and retail, will become more prevalent, offering targeted benefits.
Conclusion
In conclusion, the significance of image annotation for machine learning is unparalleled in the modern business landscape. By effectively harnessing this powerful tool, companies can enhance their products, improve customer satisfaction, and stay ahead of the competition. As technology continues to advance, businesses that prioritize investment in image annotation will find themselves at the forefront of innovation and efficiency, paving the way for a brighter future.
For more information and expert solutions in image annotation and other machine learning services, consider visiting keymakr.com to explore the opportunities that lie ahead.