Understanding the Power of Image Data Annotation
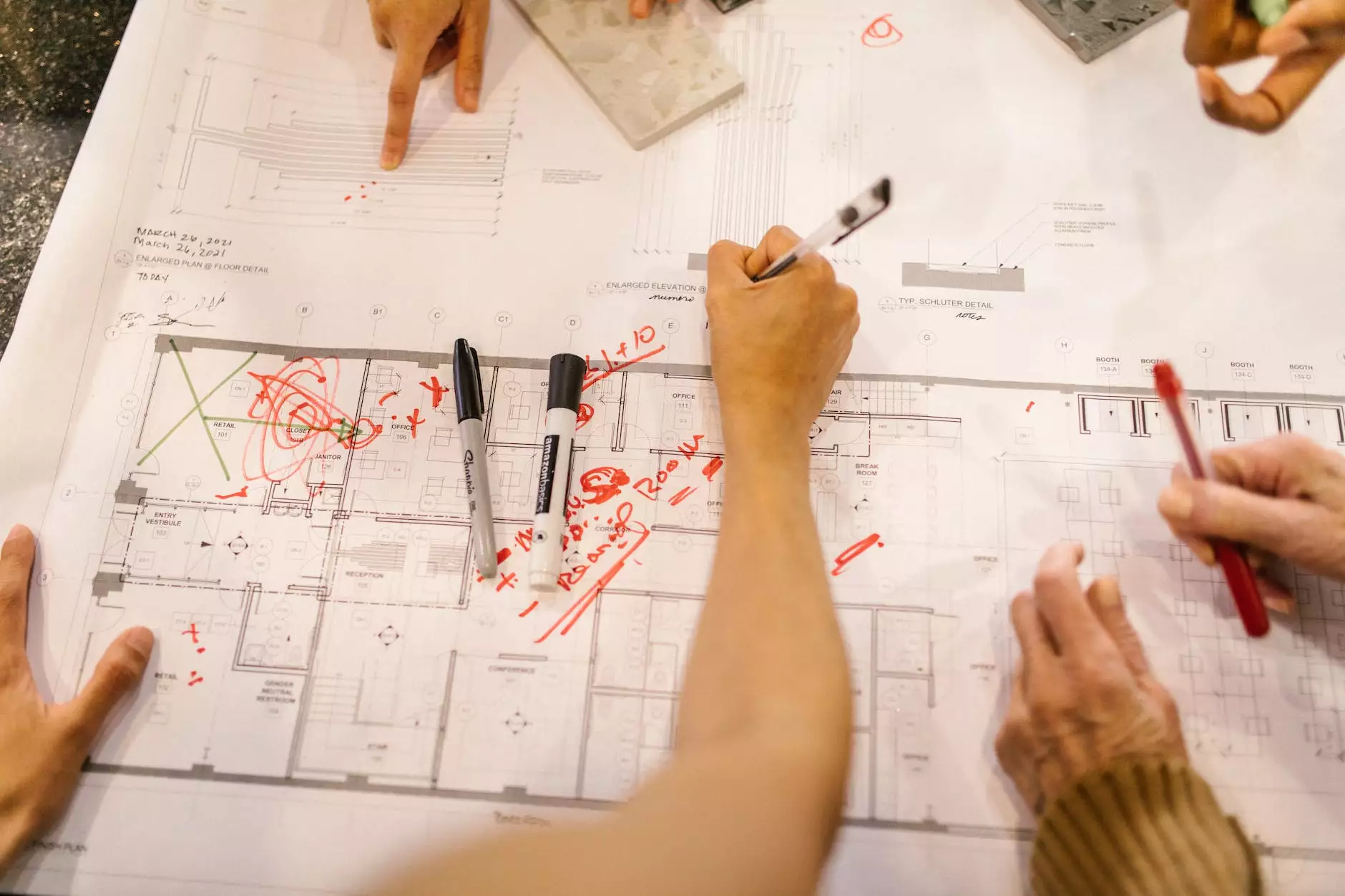
Image data annotation is more than just a simple task; it is a cornerstone of artificial intelligence (AI) and machine learning (ML). Annotation involves labeling images to train models to understand and interpret visual information effectively. As businesses increasingly leverage AI for smarter decision-making, robust data annotation solutions become paramount. This article delves into the intricacies of image data annotation, the tools available, and how to choose the right data annotation platform for your business needs.
What is Image Data Annotation?
Image data annotation is the process of tagging or labeling images to provide input for AI models, enhancing their ability to recognize patterns and make predictions based on visual data. This practice is essential in various industries such as autonomous driving, healthcare, and security. By accurately annotating images, businesses can create more reliable AI systems capable of performing tasks that require visual understanding.
Why is Image Data Annotation Important?
The importance of image data annotation cannot be overstated. Here are several reasons why it is a critical component in AI development:
- Enhances Model Accuracy: Properly annotated images help AI systems learn more accurately, leading to better predictions and outcomes.
- Facilitates Object Recognition: Automated systems can identify and classify objects within images, which is crucial in industries like retail and transportation.
- Improves Efficiency: Annotated data allows for faster processing times, reducing the time required for machine learning models to achieve high levels of accuracy.
- Supports Diverse Applications: From facial recognition to medical imaging, well-annotated datasets are vital in developing innovative applications.
The Image Data Annotation Process
The process of image data annotation can vary based on the type of data and the application needs. However, the following steps are commonly involved:
- Data Collection: Gather a large dataset of images relevant to the task.
- Annotation: Use software tools to label the images according to the defined categories, which could include bounding boxes, segmentation masks, and key points.
- Quality Assurance: Review annotated data to ensure accuracy and consistency, correcting any errors before the data is used for training.
- Integration: Utilize the annotated dataset for training machine learning models, refining them for improved accuracy and performance.
Types of Image Data Annotation Techniques
There are several techniques for image data annotation that businesses can choose from, depending on their specific needs:
- Bounding Box Annotation: This technique involves drawing a rectangular box around objects within an image, commonly used in object detection tasks.
- Semantic Segmentation: This technique segments the image into meaningful parts, labeling each pixel to define boundaries of different objects within an image.
- Instance Segmentation: Similar to semantic segmentation but capable of distinguishing between different instances of the same class, enhancing object detection capabilities.
- Keypoint Annotation: This method involves marking specific points on objects, useful in tasks such as pose estimation or facial recognition.
Choosing the Right Data Annotation Tool
When it comes to effective image data annotation, selecting the right tool is critical. Here are several factors to consider:
- User-friendliness: The tool should be intuitive and easy to use, allowing your team to annotate data efficiently.
- Supported Annotation Types: Ensure that the tool supports the annotation techniques you require for your specific project.
- Scalability: As your needs grow, the tool should be able to handle larger datasets without compromising performance.
- Collaboration Features: Look for tools that enable team collaboration, allowing multiple users to work simultaneously on different parts of the annotation process.
- Integration Capabilities: Choose a solution that can easily integrate with your existing workflows or ML platforms, streamlining the data processing pipeline.
Benefits of Using a Professional Data Annotation Platform
Utilizing a professional data annotation platform can bring numerous advantages to your business:
- Expertise: Access to experienced annotators who understand the nuances of specific tasks, ensuring high-quality labeled data.
- Time Efficiency: Speed up the annotation process significantly, allowing you to focus on model development rather than data labeling.
- Cost-Effectiveness: Outsourcing data annotation can often be more cost-effective than developing an in-house solution, particularly for small to medium-sized businesses.
- Quality Assurance: Professional platforms often incorporate thorough checks to maintain the quality and accuracy of annotated data.
Common Use Cases for Image Data Annotation
The applications of image data annotation are vast and varied. Here are some key sectors that significantly benefit from annotated images:
- Healthcare: Annotated medical imagery enhances diagnostic tools and AI-assisted imaging systems.
- Autonomous Vehicles: Accurate annotations are crucial for teaching vehicles to understand road environments and recognize obstacles.
- Retail and E-commerce: Image recognition technology can enhance shopping experiences and automate inventory management.
- Security: Surveillance systems leverage image data annotation for facial recognition and anomaly detection.
- Agriculture: Annotated imagery assists in crop monitoring and pest detection, maximizing yield and sustainability.
The Future of Image Data Annotation
As the demand for AI technology grows, so does the need for high-quality annotated datasets. The future of image data annotation is bright, driven by advancements in technology and process automation:
- AI-Assisted Annotation: New tools that utilize AI to assist human annotators can significantly reduce the time it takes to label images, improving overall productivity.
- Real-time Annotation: With advancements in streaming technology, we can expect real-time annotation capabilities for dynamic environments.
- Greater Customization: Future platforms may offer better customization options tailored to specific industry needs or unique use cases.
- Improved Collaboration: Enhanced collaborative features in annotation platforms will allow for more streamlined teamwork across geographical boundaries.
Conclusion
In summary, image data annotation plays an indispensable role in enabling artificial intelligence to function in a way that mimics human perception. The effectiveness of AI models largely hinges on the quality of the annotated data they are trained on. By adopting professional data annotation platforms like KeyLabs.ai, businesses can ensure that they remain at the forefront of technology, offering innovative solutions across various sectors. Whether you are just starting or looking to enhance existing processes, understanding and implementing effective data annotation practices is crucial for achieving AI success.
Take Action Now!
Don't let your business fall behind in the AI revolution. Contact KeyLabs.ai today and learn how our advanced data annotation tools can help you harness the full potential of your image data, transforming your AI initiatives into success stories!